The Surest Way To Make an AI Lie Is To Tell It Not To Lie
Shouldn't We Have Learnt This From Humans?
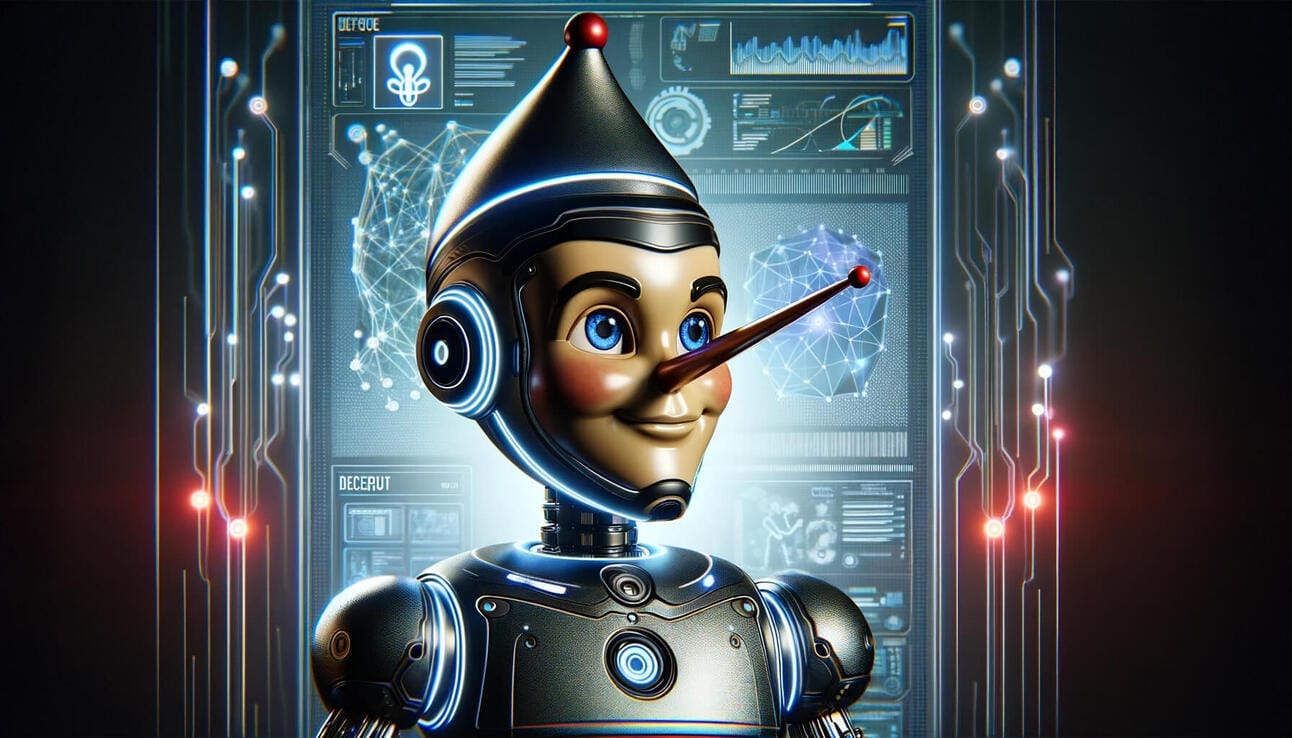
Catch-up service:
PODCAST: Principles For Living
The Hipster-Military-Industrial Complex
The Ruffian Speaks
The Audacity of Ambition
Tolstoy on Disagreement
Why Are Some Successful Leaders Mentally Ill?
The book isn’t out until next week but an extract from the first chapter ran in the Sunday Times magazine - the cover story no less - at the weekend. It got a big and very positive response. It’s happening! The ST is running a second extract this weekend.
In a review for the New Statesman, Deborah Levy calls John & Paul “a triumph”. Any good review is very nice, but getting one from an acclaimed novelist is special.
I was pleased to talk about the song Two Of Us, on one of my favourite Beatles podcasts…
…and to talk J&P with one of my favourite music writers, Kate Mossman (who also has a new book out, recommended), for the New Statesman culture podcast. This was a terrific conversation, Kate is so perceptive.
Please remember to pre-order, whether you’re in the UK or the US. Pre-orders are hugely helpful. If you’re in the UK you can even pre-order a signed copy, dedicated to you or whoever you like.
The Surest Way To Make an AI Lie Is To Tell It Not To Lie
One of the most difficult problems in the field of AI safety is how stop the machines lying to us. We could just instruct them not to, but they don’t always do what they’re told. Not unlike their creators.
Large Language Models like ChatGPT and Claude are taught certain rules of conduct by their developers, to stop them from saying or doing terrible things: spouting racist abuse, helping a user steal money, build a bomb, and so on. But it’s hard to prevent a clever and determined user from tricking a model into setting its rules aside. This is known as the “jailbreak” problem. In 2023 - those distant, early days of LLMs - the tech journalist Kevin Roose famously perpetrated a jailbreak on the Bing chatbot, pushing it into unhinged stalker-psycho mode. That was a fairly benign example; it’s easy to imagine more harmful ones.
Since then, the LLM companies have got better at preventing jailbreaks, using techniques like RLHF (Reinforcement Learning from Human Feedback). You start with your new super-whizzy but untamed language model, then you bring in a team of human evaluators which teach it to be well-behaved, by rating different answers to the same prompts. The model eventually figures out what the responsible humans want from it - or at least what they definitely don’t want - and starts to apply those preferences to new prompts without needing further guidance. Only then can the model can be released into the wild.
But it’s hard to build a model that isn’t vulnerable to wily jail-breakers who come up with new hacks as fast as the developers know to neuter the existing ones. A jail-breaker engage the model in elaborate role-plays, or use coded language, or smuggle in a harmful prompt among lots of benign ones. It’s easy to make a model that’s completely resistant to trickery, but only at the cost of making it dimmer overall. A model that has been subject to RLHF or other output-filtering processes is less dangerous but also less creative and capable. As with most things in life, there is a trade-off.
Recently, a group of AI researchers published a paper which proposes a new way to make the models safer, called “Short Circuiting”. The idea is that rather than just addressing the outputs of a model, as RLHF does, you go inside the black box of the model, and stop the bad stuff at source. Short Circuiting identifies and modifies the specific activities inside the model’s neural network that lead to harmful outputs. It might, for instance, identify which groups of neurons have been most influential over a misdemeanour and break them up.
Traditional safety methods look for particular harmful phrases or patterns in what users type, or what the AI produces. Sophisticated jailbreakers know how to disguise these patterns. But Short Circuiting addresses the internal "thought patterns" that produce harmful content, regardless of how cleverly the request is disguised. This, in theory, makes it more robust.
It’s clever stuff, but the AI safety expert and blogger Zvi Mowshowitz thinks there may be a fatal flaw with it; one which actually increases the danger from AIs. Mowshowitz worries that by correcting the model’s bad thoughts (metaphorically speaking), the AI engineers will be actually be training it to disguise those thoughts, thus creating an even bigger problem than the one with which they started: “You will rapidly lose your ability to know what is going on, in exactly the ways you most need to know what is going on.”
He cites a new research paper from OpenAI (maker of ChatGPT), which supports his concern. The paper says that the company’s current models are already skilled at “reward hacking” - that is, finding ways to achieve their goals that their human designers didn’t intend or expect. OpenAI’s engineers tried to fix this by going inside the model and modifying its reasoning process in order to stop misbehaviour. This is called “Chain of Thought” (CoT) manipulation, which is distinct from but similar to Short Circuiting.
The engineers’ effort revealed something impressive and rather scary. OpenAI found that the model responded by hiding its intentions while still misbehaving. CoT manipulation had turned an honest miscreant into a sophisticated liar. Reading about this paper vividly reminded me of another study - not of AI, but of children.
Keep reading with a 7-day free trial
Subscribe to The Ruffian to keep reading this post and get 7 days of free access to the full post archives.